Investigating Netflix Movies and Guest Stars in The Office
1. Welcome!
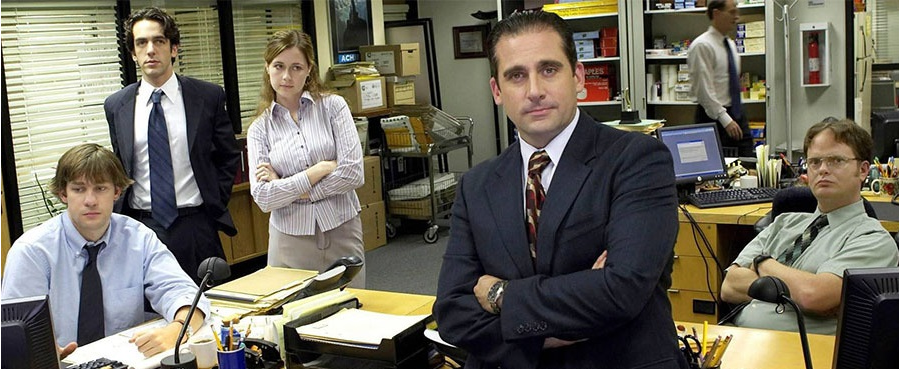
The Office! What started as a British mockumentary series about office culture in 2001 has since spawned ten other variants across the world, including an Israeli version (2010-13), a Hindi version (2019-), and even a French Canadian variant (2006-2007). Of all these iterations (including the original), the American series has been the longest-running, spanning 201 episodes over nine seasons.
In this notebook, we will take a look at a dataset of The Office episodes, and try to understand how the popularity and quality of the series varied over time. To do so, we will use the following dataset: datasets/office_episodes.csv, which was downloaded from Kaggle here.
This dataset contains information on a variety of characteristics of each episode. In detail, these are:
datasets/office_episodes.csv
episode_number: Canonical episode number.
season: Season in which the episode appeared.
episode_title: Title of the episode.
description: Description of the episode.
ratings: Average IMDB rating.
votes: Number of votes.
viewership_mil: Number of US viewers in millions.
duration: Duration in number of minutes.
release_date: Airdate.
guest_stars: Guest stars in the episode (if any).
director: Director of the episode.
writers: Writers of the episode.
has_guests: True/False column for whether the episode contained guest stars.
scaled_ratings: The ratings scaled from 0 (worst-reviewed) to 1 (best-reviewed).
First we have to import the pandas and matplotlib libraries
import pandas as pd
import matplotlib.pyplot as plt
plt.rcParams['figure.figsize'] = [11, 7]
Read the csv file as DataFrame office_df and display the first five rows.
office_df = pd.read_csv('datasets/office_episodes.csv')
office_df.head()
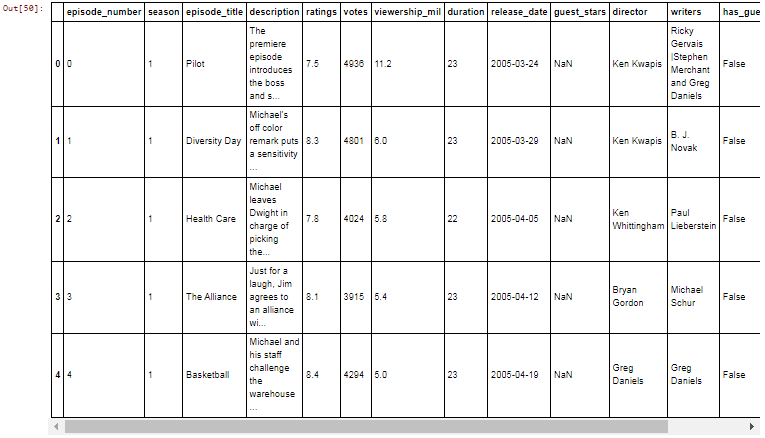
For data visualisation we define colors based on the given statements to reflect the different ratings
cols =[]
for i, row in office_df.iterrows():
if row['scaled_ratings'] < 0.25:
cols.append('red')
elif row['scaled_ratings'] < 0.50:
cols.append('orange')
elif row['scaled_ratings'] < 0.75:
cols.append('lightgreen')
else:
cols.append('darkgreen')
Sizing the guest appearance with marker size 250 and those without guest marker size as 25, as per the instruction
size = []
for i, row in office_df.iterrows():
if row['has_guests']==True:
size.append(250)
else:
size.append(25)
We create columns for color and size also define DataFrame for with guest and without guest
office_df['colors']=cols
office_df['size']=size
with_guest_df = office_df[office_df['has_guests'] == True]
no_guest_df = office_df[office_df['has_guests'] == False]
For data visualization
fig=plt.figure()
plt.style.use('fivethirtyeight')
plot_1=plt.scatter(data=no_guest_df,x="episode_number",y="viewership_mil",c='colors',s='size')
plot_2=plt.scatter(data=with_guest_df,x="episode_number",y="viewership_mil",c='colors',s='size',marker='*')
We add title, xlabel and ylabel. And display the plot
plt.title("Popularity, Quality, and Guest Appearances on the Office")
plt.xlabel("Episode Number")
plt.ylabel("Viewership (Millions)")
plt.show()
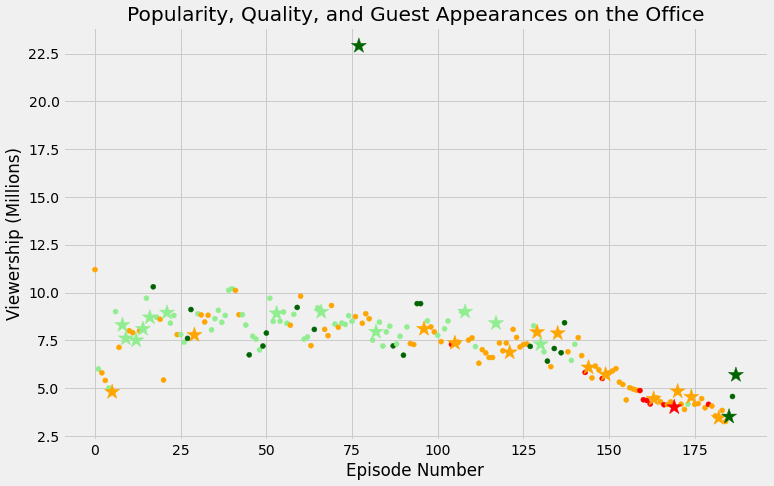
Now to get the most watched episode
office_df_most_watched=office_df[office_df['viewership_mil']==office_df['viewership_mil'].max()]
To view the top star person
# to view the top star persontop_stars=office_df_most_watched['guest_stars']top_stars
#OUTPUT
Cloris Leachman, Jack Black, Jessica Alba
Name: guest_stars, dtype: object
Comments